The two most disruptive political events of the last few years are undoubtedly the Brexit referendum to leave the European Union and the election of Donald Trump. Both are commonly associated with the political consulting firm Cambridge Analytica and a technique known as Microtargeting.
If you want to understand the data science behind the Cambridge Analytica/Facebook data scandal and Microtargeting (i.e. LASSO regression) by building a toy example in R read on!
The following post is mainly based on the excellent case study “Cambridge Analytica: Rise and Fall” by my colleague Professor Oliver Gassmann (who was so kind as to email it to me) and Raphael Boemelburg, both from the University of St. Gallen, Switzerland (where I did my PhD), and “Weapons of Micro Destruction: How Our ‘Likes’ Hijacked Democracy” by Data Scientist Dave Smith (the data for the toy example is also from that article).
Also well worth a watch is the Netflix documentary “The Great Hack”. I encourage all of my colleagues from academia and all teachers to consider screening it to their students/pupils. Not many know that Netflix is kind enough to provide a license to do this legally: Neflix grant of permission for educational screenings. In this documentary, it becomes clear that microtargeting can be considered as some form of psychological warfare and information warfare, i.e. as a military-grade weapon with the potential to destroy our democratic system:
So, how does it actually work?
Basically, Microtargeting is the prediction of psychological profiles on the basis of social media activity and using that knowledge to address different personality types with customized ads. Microtargeting is not only used in the political arena but of course also in Marketing and Customer Relationship Management (CRM).
A well-known psychological model is the so-called OCEAN model:
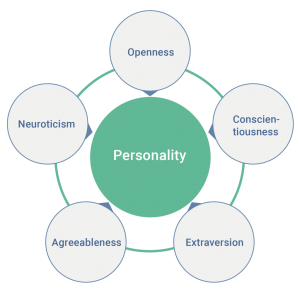
The five personality traits are:
- Openness to experience (inventive/curious vs. consistent/cautious)
- Conscientiousness (efficient/organized vs. easy-going/careless)
- Extraversion (outgoing/energetic vs. solitary/reserved)
- Agreeableness (friendly/compassionate vs. challenging/detached)
- Neuroticism (sensitive/nervous vs. secure/confident)
You can find out about your own personality by taking this free, anonymous test: The Big Five Project Personality Test.
If you had the psychological profiles of many individuals you could use this together with modern advertising technology (which lets you show different ads to different people) to cater to their individual needs with the aim to manipulate them very efficiently:
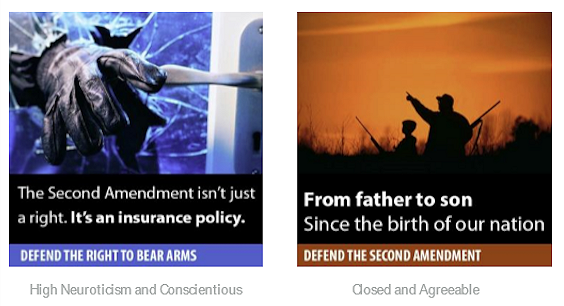
So far, so standard… the difficult part is predicting psychological traits with high accuracy! And here comes data science into play, namely a technique called LASSO regression (for Least Absolute Shrinkage and Selection Operator), or simply the LASSO.
If you are not familiar with Classical Linear Regression (CLR) please read my post Learning Data Science: Modelling Basics first. The difference between CLR and the LASSO is that with the latter you simultaneously minimize the error of the regression and the sum of the coefficients so that some coefficients will become zero. Thereby you only retain the important variables, so LASSO regression provides automatic variable selection! The other effect is that by effectively reducing the complexity of the model (also called shrinkage in this context, some form of regularization) you prevent overfitting.
Mathematically you minimize the following expression:
The first summand is the error term of classical linear regression (the difference between the real values and the predicted values), the second is the regularization term.
(lambda) is a hyperparameter which controls how big the shrinkage of the coefficients get (the bigger
the smaller the
coefficients).
Enough of the theory, let’s get to a toy example! We use the psychological factor “Openness” as the trait to predict (Facebook got those data by personality tests which people could take). We have seven individuals with their openness score and their individual likes on certain Facebook posts (you can find the data for this example here: cambridge-analytica.csv). We use five for the training set and two for the test set:
data <- read.csv("data/cambridge-analytica.csv") data ## Openness Person The_Colbert_Report TED George_Takei Meditation ## 1 1.85 Adam 1 1 1 1 ## 2 1.60 Bob 1 1 1 1 ## 3 -0.26 Cathy 0 1 1 0 ## 4 -2.00 Donald 0 1 0 0 ## 5 -2.50 Erin 0 0 0 0 ## 6 1.77 Hilary 1 1 1 1 ## 7 -2.20 Mike 0 0 0 0 ## Bass_Pro_Shops NFL_Network The_Bachelor ## 1 0 0 0 ## 2 0 0 0 ## 3 0 0 1 ## 4 1 1 0 ## 5 1 1 1 ## 6 0 0 0 ## 7 1 1 0 ## Ok_If_we_get_caught_heres_the_story ## 1 0 ## 2 1 ## 3 1 ## 4 1 ## 5 1 ## 6 1 ## 7 1 x_train <- as.matrix(data[1:5, 3:10]) x_test <- as.matrix(data[6:7, 3:10]) y_train <- as.matrix(data[1:5, 1]) y_test <- as.matrix(data[6:7, 1])
Now we build the actual model with the excellent glmnet
package (on CRAN), which has been co-developed by one of the discoverers of the LASSO, my colleague Professor Robert Tibshirani from Standford University, and after that plot how the coefficients get smaller the bigger gets:
library(glmnet) ## Loading required package: Matrix ## Loading required package: foreach ## Loaded glmnet 2.0-18 LASSO <- glmnet(x_train, y_train, alpha = 1) # alpha = 1 for LASSO regression plot(LASSO, xvar = "lambda") legend("topright", lwd = 1, col = 1:6, legend = colnames(x_train), cex = .7)
In the plot, you can see how growing lets the coefficients shrink. To find a good value for
we use a technique called cross-validation. What it basically does is building a lot of different training- and test-sets automatically and averaging the error over all of them for different values of
. After that, we plot the resulting errors with upper and lower standard-deviations:
cv_LASSO <- cv.glmnet(x_train, y_train) ## Warning: Option grouped=FALSE enforced in cv.glmnet, since < 3 observations ## per fold plot(cv_LASSO)
In this case, we see that the minimal gives the smallest error, so we choose it for the prediction of the openness score of our test set:
round(predict(LASSO, x_test, s = cv_LASSO$lambda.min), 2) ## 1 ## 6 1.58 ## 7 -2.41 y_test ## [,1] ## [1,] 1.77 ## [2,] -2.20
Not too bad! In reality, with only 70 likes the algorithm can assess personality better than a friend of the person would be able to, with 150 likes it is better than the parents, and with 300 likes, it is even better than the spouse! Creepy, isn’t it!
Another big advantage is the interpretability of LASSO regression. It is easily discernible that “The Colbert Report” and “George Takei” (a former Star Trek actor who became a gay rights and left-leaning political activist) are the biggest drivers here:
round(coef(LASSO, s = cv_LASSO$lambda.min), 2) ## 9 x 1 sparse Matrix of class "dgCMatrix" ## 1 ## (Intercept) -2.23 ## The_Colbert_Report 1.84 ## TED 0.43 ## George_Takei 1.72 ## Meditation 0.00 ## Bass_Pro_Shops . ## NFL_Network . ## The_Bachelor . ## Ok_If_we_get_caught_heres_the_story -0.18
Another advantage is that you need much fewer data points to produce accurate predictions because many attributes drop out (i.e. their coefficients become zero).
It is no overstatement to say that with those new possibilities of microtargeting we have entered a new era of potential (and real!) manipulation. I hope that you now understand the data science behind it better.
In my opinion that knowledge is important to be part of the necessary conversation about the consequences for our society. This conversation has only just begun… looking forward to your comments on this topic!
I think your post is unnecessarily alarmist. In this whole story, the “difficult part” is NOT “predicting psychological traits with high accuracy”, the difficult part is to not just “aim to manipulate [voters] very efficiently”, but to actually do so. Who knows whether microtargeting has any significant effect on voting behavior? This part is complete speculation.
Also, if you consider the vast -and overt – bias in media reporting, there you have something to worry about.
You are right, the absolute effect is hard to determine but keep in mind that both the Brexit referendum and Trump’s election were effectively too close to call and I would be very surprised if bombarding voters with hundreds of millions of psychologically customized ads had had no effect…
“…the difficult part is to not just “aim to manipulate [voters] very efficiently”, but to actually do so. Who knows whether microtargeting has any significant effect on voting behavior?…”
The entire history of marketing science, psychology, sociology, economics, etc… would disagree. This is about marketing. This is about impressions. This is about finding images/ads that resonate with people. Why do think billions of dollars are spent on marketing if marketing doesn’t change behavior? You really think there are that many people in the world riding mountain bikes off a cliff to sustain Go-Pro. They are massively successful at marketing a lifestyle, not a camera, because people see their ads and think “I can be like that if I had a Go-Pro.”
Does any one given ad, seen one time, change behavior in a dramatic way? Almost certainly not. If I’m a 2nd amendment proponent and registered democrat, seeing one ad, one time, isn’t going to swing me to a republican vote. But, if I’m high on neuroticism/anxiety, and I see the ad on the left, time and time and time and time again, in various iterations, over and over and over, then things get a little more interesting. The ad starts to resonate with me. Now the message starts to trigger (e.g. amplify) my own anxieties. And I can’t get away from these kinds of ads, because everywhere I go online I keep seeing things like this. And now, my own sentiments and voting proclivities may start to change. Hammer these things away at the middle of the electorate and it’s not hard to see how things would swing one way or the other, especially in closer elected contests.
The only thing that is new now is the scale, ease, and relatively inexpensive ways targeted advertising can be deployed.
For me, the more difficult part would have been getting accurate personality profiles on people to begin with. You need to correctly classify them in the first place before you can target them. The rest is child’s play as it’s just predictive modeling of one sort or another. But then seeing how people eat up these online “personality” tests through buzzfeed, the amount of web-tracking/web-scraping that goes on, and the like, even that doesn’t seem so insurmountable anymore.
jeff: Thank you for your comment: I think you have presented the whole matter in a very impressive and easy-to-understand way!
Professor, thanks for the article. Great job, clear and accurate.
Thank you for your feedback!
“Research from the Universidad Carlos III de Madrid (UC3M), the University of Warwick and ETH Zurich has studied the effectiveness of micro-targeted political advertising on social media such as Facebook in the United States. The research concludes that it may have increased the number of Donald Trump voters by ten per cent in the 2016 presidential elections.”
https://www.uc3m.es/ss/Satellite/UC3MInstitucional/en/Detalle/Comunicacion_C/1371258998853/1371216001259/A_study_analyses_the_impact_of_targeted_Facebook_advertising_on_the_elections
Very interesting, didn’t know that – Thank you!
So far as the effectiveness: Trump was ‘elected’ by 78,900 votes in three states (PA, MI, WI), in particular in the least educated districts. Or as Trump has said, “I love the poorly educated”. One needn’t be of the Eastern Effete Educated Elite to understand that the manipulation described here simply works better on those with the least level higher-order thinking. His margin was razor thin and unexpected even by him.
Corporates have used ‘market segmentation’ forever seeking to drain consumer’s surplus. This is really no different. Much of the data science workforce is engaged in ‘market segmentation’ implementation.
I agree, the general principle is the same. What is new though is that now it can be done automatically on a micro-level, thereby creating a personalized reality for every citizen/customer others cannot perceive, even if they wanted to. That makes the whole thing so effective and so dangerous.
Let’s be clear: what’s presented here by Jeff and others is still just a story of how things might happen. Yes, while it may sound plausible that targeting effects are small by themselves, but significantly stronger when cumulated, it may also not be.
But the really important point is that the dominant political-ideological climate that has been growing stronger and stronger in the US over the past decades, possessing not only universities, but the mainstream media and tech, has openly and constantly been bombarding society with the canons of political correctness, SJW thinking, oppression and victimhood mentality, virtue signalling, anti white-male rhetoric etc. If you want to worry about voter influencing, here’s the gigantic elephant in the room.
Finally, you wrote that Trump’s election and the Brexit referendum were the most disruptive political events in recent time. I know what you mean but this is not an accurate description. In both cases it is the hysterical reaction (in the case of Trump by the left) to these results of a democratic election that was disruptive. That’s the proper subject matter here.
To be honest with you, I don’t get it: on the one hand, you bemoan the “dominant political-ideological climate” which you consider to be left-wing. On the other hand, you doubt that the social media strategy used by the Trump campaign had any significant impact…
My question for you: if the dominant (!) climate was really so Anti-Trump and the social media strategy of his campaign didn’t work why was he elected anyway?
Just as an aside concerning the so-called mainstream media: “As of September 2019, Fox News was the most-watched network for the third consecutive year and continues to do well in terms of its prime time audience, with around 2.43 million primetime viewers in that month.” (Source: statista.com). Next comes MSNBC with 1.5 million and CNN with just over 1 million… so much for “mainstream”.
My point is that you got your priorities wrong if you worry only about the effect of one relatively small, particular manipulation when there is large-scale manipulation at universities and by the media going on all the time.
Re: dominant ideology, I should have been more precise: left-wing views are dominant in the media, in humanities programs at colleges and universities, and in big tech and other companies. In terms of number of voters, right-and left-wing views are roughly equal, as we could see in teh 2016 election. But the PC fraction of the left has a disproportionate influence in the public sphere.
” oppression and victimhood mentality”
These were/are the core elements of both Trump (and his right wing cabal) and Brexit, not the so-called left. Exploiting the rural, uneducated, low income rural white folk who consider themselves oppressed is how both came to be. The simple fact is that the rural, uneducated, low income white folk in both countries ended up that way because they insist on electing those who begrudge them education, healthcare, and the like. One only read the ravings of Johnson and Trump to see their victimhood. There’s a reason that the marginally above median smart young folk get the hell out of rural and migrate to cities. One side effect of being urban is exposure to multi-culti, and a general (if not universal) waning of insular prejudice.
Not long ago I let my fingers do the walking through the Yellow Googles, to discover how many US states were majority rural. All but Maine, Mississippi, Vermont, and West Virginia are majority urban. The Trump/Brexit folks have only a short window of opportunity to institute ‘the final election’ of their oppressed white men.